September 20 – 22, 2021
Videos and Slides:
Slides:
- Ricardo Baeza-Yates: Ethics in AI: A Challenging Task
- Stephanie Law: Anti-discrimination Law and AI-based DM
- Hinrich Schuetze: Humans Learn From Task Descriptions and So Should Our Models
- Katharina Kinder-Kurlanda: AI and Research Ethics – Theory & Practice
Videos:
- Steffen Staab Welcome
- Krishna Gummadi Foundations for Fair Algorithmic Decision Making
- Ricardo Baeza-Yates Ethics in AI: A Challenging Task
- Stephanie Law The Procedural Obstacles of Enforcing Anti-discrimination Law in the Context of AI-based Decision-making
- Mathias Niepert Fairness and Bias in Machine Learning
- Katharina Kinder-Kurlanda AI and Research Ethics – Theory & Practice
Registration
You can register for one, two, or all three days. To listen to the keynotes and lectures for the various days, please register here:
See the detailed schedule below to learn about who is speaking at the individual sessions. The topics of the lectures and keynotes can be found with the information about each speaker.
Please check back regularly as we are continually updating this information.
Lunch Break
During the lunch break, you are invited to come and discuss the lectures and keynotes in the NoBIAS Lunch Room.
Schedule
Monday, Sept. 20, 2021
9:30 – 18:30 CEST (8:30 – 17:30 BST)
- 09:30 – 10:00 Opening and Welcome
- 10:00 – 11:00 Keynote by Krishna Gummadi
Foundations for Fair Algorithmic Decision Making
-
- Break
- 11:30 – 13:00 Lecture by Ricardo Baeza-Yates
Ethics in AI: A Challenging Task
- 13:00 – 14:30 Lunch break – NoBIAS Lunch Room. Open to everyone to come and discuss the lectures and keynote!
- 14:30 – 16:30 Workshop*
- Break
- 17:00 – 18:00 Keynote by Alexandra Olteanu
Failures of Imagination: Challenges to the Discovery and Measurement of Computational Harms
Tuesday, Sept. 21, 2021
9:30 – ca. 16:00 CEST (8:30 – ca. 15:00 BST)
- 9:30 – 11:00 Lecture by Stephanie Law
The Procedural Obstacles of Enforcing Anti-discrimination Law in the Context of AI-based Decision-making
-
- Break
- 11:30 – 13:00 Lecture by Mathias Niepert
Fairness and Bias in Machine Learning
- 13:00 – 14:30 Lunch break – NoBIAS Lunch Room. Open to everyone to come and discuss the lectures and keynote!
- 14:30 – open-end Workshop*
Wednesday, Sept. 22, 2021
9:30 – 17:00 CEST (8:30 – 16:00 BST)
- 9:30 – 10:30 Keynote by Hinrich Schuetze
Humans Learn From Task Descriptions and So Should Our Models
Joint work with Timo Schick and Sahana Udupa
-
- Break
- 11:00 – 12:30 Lecture by Katharina Kinder-Kurlanda
AI and Research Ethics – Theory & Practice
- 12:30 – 14:00 Lunch break
- 13:00 – 13:30 NoBIAS Lunch Room. Open to everyone to come and discuss the lectures and keynote!
- 13:00 – 13:30 NoBIAS Lunch Room. Open to everyone to come and discuss the lectures and keynote!
- 14:00 – 15:30 Reports from the workshops*
- Break
- 16:00 – 17:00 Keynote by Annette Zimmermann
The Power of Choosing Not to Build: Justice, Non-Deployment, and the Purpose of AI Optimization
- 17:00 – 17:15 Closing
Keynotes
Krishna Gummadi
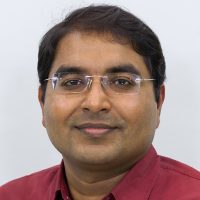
Date and Time:
- Monday, September 20, 2021, at 10:00 CEST
- To listen to this talk, make sure you have registered for the Monday Session.
Title:
Foundations for Fair Algorithmic Decision Making
Abstract:
Algorithmic (data-driven learning-based) decision making is increasingly being used to assist or replace human decision making in a variety of domains ranging from banking (rating user credit) and recruiting (ranking applicants) to judiciary (profiling criminals) and journalism (recommending news-stories). Recently concerns have been raised about the potential for discrimination and unfairness in such algorithmic decisions. Against this background, in this talk, I will attempt to address the following foundational questions about algorithmic unfairness:
(a) How do algorithms learn to make unfair decisions?
(b) How can we quantify (measure) unfairness in algorithmic decision making?
(c) How can we control (mitigate) algorithmic unfairness? i.e., how can we re-design learning mechanisms to avoid unfair decision making?
Bio:
Krishna Gummadi is a scientific director and head of the Networked Systems research group at the Max Planck Institute for Software Systems (MPI-SWS) in Germany. He also holds a professorship at the University of Saarland. He received his Ph.D. (2005) and B.Tech. (2000) degrees in Computer Science and Engineering from the University of Washington and the Indian Institute of Technology, Madras, respectively.
Krishna’s research interests are in the measurement, analysis, design, and evaluation of complex Internet-scale systems. His current projects focus on understanding and building social computing systems, with an emphasis on enhancing fairness and transparency of machine (data-driven and learning-based) decision making in such systems.
Krishna’s work on fair machine learning, online social networks and media, Internet access networks, and peer-to-peer systems has been widely cited and his papers have received numerous awards, including Test of Time Awards at ACM SIGCOMM and AAAI ICWSM, Casper Bowden Privacy Enhancing Technologies (PET) and CNIL-INRIA Privacy Runners-Up Awards, IW3C2 WWW Best Paper Honorable Mention, and Best Papers at NIPS ML & Law Symposium, ACM COSN, ACM/Usenix SOUPS, AAAI ICWSM, Usenix OSDI, ACM SIGCOMM IMC, ACM SIGCOMM CCR, and SPIE MMCN. He has also co-chaired AAAI’s ICWSM 2016, IW3C2 WWW 2015, ACM COSN 2014, and ACM IMC 2013 conferences. He received an ERC Advanced Grant in 2017 to investigate “Foundations for Fair Social Computing”.
Back to the schedule
Alexandra Olteanu
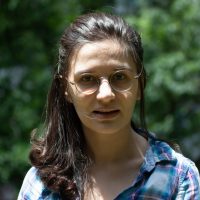
Date and Time:
- Monday, September 20, 2021, at 17:00 CEST
- To listen to this talk, make sure you have registered for the Monday Session.
Title:
Failures of Imagination: Challenges to the Discovery and Measurement of Computational Harms
Abstract:
There is a rich and long-standing literature on detecting and mitigating a wide range of biased, objectionable, or deviant content and behaviors, including hateful and offensive speech, misinformation, and discrimination. There is also a growing literature on fairness, accountability, and transparency in computational systems that is concerned with how such systems may inadvertently engender, reinforce, and amplify such behaviors. While many systems have become increasingly proficient at identifying clear cases of objectionable content and behaviors—by both humans and machines—many challenges still persist.
While existing efforts tend to focus on issues that we know to look for, techniques for preempting future issues that may not yet be on the product teams’ and research community’s radar are not nearly as well developed or understood. Addressing this gap requires deep dives into specific application areas. Current approaches to quantifying computational harms also often embed many unnamed assumptions, with poorly understood implications to the fairness and inclusiveness of a system. I will ground our discussion in some of our recent research examining how language technologies are being evaluated and how we could do better.
Bio:
Alexandra Olteanu is a principal researcher at Microsoft Research Montréal, part of the Fairness, Accountability, Transparency, and Ethics (FATE) group. Her work currently examines practices and assumptions made when evaluating a range of computational systems, particularly measurements aimed at quantifying possible computational harms. Before joining Microsoft Research, Alexandra was a Social Good Fellow at IBM’s T.J. Watson Research Center. Her work has been featured in governmental reports and in popular media outlets. Alexandra has co-organized tutorials/workshops and has served on the program committee of all major web and social media conferences, including SIGIR, ICWSM, KDD, WSDM, WWW, as the Tutorial Co-chair for ICWSM 2018, 2020 and FAccT 2018. She also sits on the steering committee of the ACM Conference on Fairness, Accountability, and Transparency. Alexandra holds a Ph.D. in Computer Science from École Polytechnique Fédérale de Lausanne (EPFL), Switzerland.
Back to the schedule
Hinrich Schuetze
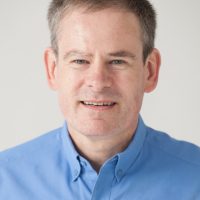
Date and Time:
- Wednesday, September 22, 2021, at 9:30 CEST
- To listen to this talk, make sure you have registered for the Wednesday Session.
Title:
Humans Learn From Task Descriptions and So Should Our Models
Joint work with Timo Schick and Sahana Udupa
Abstract:
Task descriptions are ubiquitous in human learning. They are usually accompanied by a few examples, but there is little human learning that is based on examples only. In contrast, the typical learning setup for NLP tasks lacks task descriptions and is supervised with 100s or 1000s of examples.
We introduce Pattern-Exploiting Training (PET), an approach to learning that mimics human learning in that it leverages task descriptions in few-shot settings. PET is built on top of a pre-trained language model that “understands” the task description, especially after finetuning, resulting in excellent performance compared to other few-shot methods. In particular, a model trained with PET outperforms GPT-3 even though it has 99.9% fewer parameters.
The idea of task descriptions can also be applied to reducing bias in text generated by language models. Instructing a model to reveal and reduce its biases is remarkably effective as I will show in an evaluation on several benchmarks. This may contribute in the future to a fairer and more inclusive NLP.
Bio:
Hinrich Schuetze is Professor for Computational Linguistics and director of the Center for Information and Language Processing at the University of Munich (LMU Munich). Before moving to Munich in 2013, he taught at the University of Stuttgart from 2004 to 2012. He worked on natural language processing and information retrieval technology at Xerox PARC, at several Silicon Valley startups and at Google 1995-2004 and 2008/9. After studying computer science and mathematics at Technical University Braunschweig and University of Stuttgart, he received his PhD in computational linguistics from Stanford University in 1995.
He is a coauthor of Foundations of Statistical Natural Language Processing (MIT Press 1999, with Chris Manning) and Introduction to Information Retrieval (Cambridge University Press 2008, with Chris Manning and Prabhakar Raghavan). He received an Opus Magnum Grant from Volkswagenstiftung in 2015 and a European Research Council Advanced Grant (NonSequeToR: Non-sequence models for tokenization replacement) in 2017. In 2020, he was president of the Association for Computational Linguistics. Ever since starting his PhD in the early 1990s, Hinrich’s research interests have been at the interface of linguistics, neural networks and computer science.
Back to the schedule
Annette Zimmermann
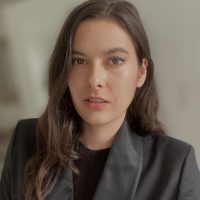
Date and Time:
- Wednesday, Sept. 22, 2021, at 16:00 CEST
- To listen to this talk,make sure you have registered for the Wednesday Session.
Title:
The Power of Choosing Not to Build: Justice, Non-Deployment, and the Purpose of AI Optimization
Abstract:
Are there any types of AI that should never be built in the first place? The ‘Non-Deployment Argument’—the claim that some forms of AI should never be deployed, or never even built—has been subject to significant controversy recently: non-deployment skeptics fear that it will stifle innovation, and argue that the continued deployment and incremental optimization of AI tools will ultimately benefit everyone in society. However, there are good reasons to subject the view that we should always try to build, deploy, and gradually optimize new AI tools to critical scrutiny: in the context of AI, making things better is not always good enough. In specific cases, there are overriding ethical and political reasons—such as the ongoing presence of entrenched structures of social injustice—why we ought not to continue to build, deploy, and optimize particular AI tools for particular tasks. Instead of defaulting to optimization, we have a moral and political duty to critically interrogate and contest the value and purpose of using AI in a given domain in the first place.
Bio:
Dr. Annette Zimmermann is a political philosopher working on the ethics of algorithmic decision-making, machine learning, and artificial intelligence. Additional research interests include moral philosophy (particularly the ethics of risk and uncertainty) and legal philosophy (the philosophy of punishment), as well as the philosophy of science (models, explanation, abstraction). Zimmermann’s current research project (“The Algorithmic Is Political”) explores how disproportionate distributions of risk and uncertainty associated with the use of emerging technologies like AI and machine learning impact democratic values like equality and justice.
Zimmermann is a permanent Lecturer (US equivalent: Assistant Professor) in Philosophy at the University of York, and they conducted their postdoctoral research at Princeton University. Zimmermann holds a DPhil (PhD) and MPhil from the University of Oxford (Nuffield College and St Cross College), as well as a BA from the Freie Universität Berlin. They have held visiting positions at Stanford University, the Australian National University, Yale University, and SciencesPo Paris. They have worked with policy-makers at the OECD, UNESCO, the UK Parliament, the Australian Human Rights Commission, the German Aerospace Center and the German Federal Ministry for Economic Affairs and Energy, as well as the UK Centre for Data Ethics and Innovation.
Back to the schedule
Lectures
Ricardo Baeza-Yates
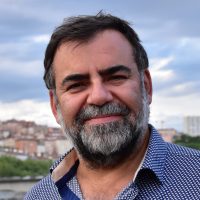
Date and Time:
- Monday, September 20, 2021, at 11:30 CEST
- To listen to this lecture, make sure you have registered for the Monday Session.
Title:
Ethics in AI: A Challenging Task
Abstract:
In the first part we cover five current specific challenges through examples emphasizing bias issues: (1) discrimination (e.g., facial recognition, justice, sharing economy, language models); (2) phrenology (e.g., biometric based predictions); (3) unfair digital commerce (e.g., exposure and popularity bias); (4) stupid models (e.g., lack of understanding semantics and context) and (5) indiscriminated use of computing resources (e.g., large language models). These examples do have a personal bias but set the stage for the second part where we address four generic challenges: (1) too many principles (e.g., principles vs. techniques), (2) cultural differences (e.g., Christian vs. Muslim); (3) regulation (e.g., privacy, antitrust) and (4) our cognitive biases. We finish discussing what we can do to address these challenges in the near future.
Bio:
Ricardo Baeza-Yates is Director of Research at the Institute for Experiential AI of Northeastern University. He is also part-time professor at Universitat Pompeu Fabra in Barcelona and Universidad de Chile in Santiago. Before, he was VP of Research at Yahoo Labs, based in Barcelona, Spain, and later in Sunnyvale, California, from 2006 to 2016. He is co-author of the best-seller Modern Information Retrieval textbook published by Addison-Wesley in 1999 and 2011 (2nd ed), that won the ASIST 2012 Book of the Year award. From 2002 to 2004 he was elected to the Board of Governors of the IEEE Computer Society and between 2012 and 2016 was elected for the ACM Council. Since 2010 is a founding member of the Chilean Academy of Engineering. In 2009 he was named ACM Fellow and in 2011 IEEE Fellow, among other awards and distinctions. He obtained a Ph.D. in CS from the University of Waterloo, Canada, in 1989, and his areas of expertise are web search and data mining, information retrieval, bias on AI, data science and algorithms in general.
Back to the schedule
Stephanie Law
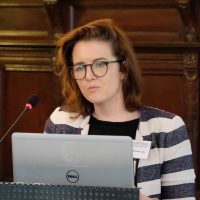
Date and Time:
- Tuesday, Sept. 21, 2021, at 9:30 CEST
- To listen to this lecture, make sure you have registered for the Tuesday Session.
Title:
The Procedural Obstacles of Enforcing Anti-discrimination Law in the Context of AI-based Decision-making
Abstract:
It seems trite to state that AI-based decision making poses challenges to fundamental rights, and in particular to the principles of non-discrimination and equality. These consequences of AI-based decision making have been recognised in various studies and by numerous institutions including, amongst others, the European Commission. In the preamble to its White Paper on Artificial Intelligence, the Commission highlights that AI “entails a number of potential risks including gender-based or other kinds of discrimination”; a similar acknowledgment is made in the Commission’s most recent legislative proposal on AI. The various biases at the centre of the research undertaken within the NoBias project are deemed to be at the core of these interferences with anti-discrimination and equality law.
Given the breadth of the threats posed by AI-based decision making, it is almost impossible to deal with all challenges coherently or in satisfactory depth in a single presentation. Instead, this presentation focuses on a set of procedural law challenges. It begins by establishing the current legal framework of anti-discrimination law and proceeds to identify and explain briefly four key challenges posed by AI-based decision making: the omnipresence of AI-based decision making and limited fields in which non-discrimination law might apply; the lacking scope of non-discrimination law to deal with intersectional discrimination, the relationship between direct and indirect discrimination and finally, whether non-discrimination law can be enforced satisfactorily to address the threats of AI-based decision making. This research delves further into the last challenge, and from the perspective of procedural justice, elaborates on the key questions raised as regards whether the legal framework is fit for purpose and what changes are necessary. These questions include: what type (legal) action can be brought? Before which legal forum? What is the substance of the claim and what difficulties arise from establishing discrimination arising from AI? How to fund a claim? What remedies are available and how can they be enforced?
Bio:
Since 2019, Stephanie Law has been a lecturer at Southampton Law School at the University of Southampton where she teaches in the fields of international adjudication and private international law. Between 2015 and 2019, she was a Senior Research Fellow at the Max Planck Institute Luxembourg for Procedural Law. She is a graduate of the University of Glasgow (LL.B., First Class, 2009), the University of Edinburgh (LL.M., Distinction, 2010) and the European University Institute in Florence (Ph.D., 2014). Prior to joining the MPI, she was a Leverhulme Trust-funded postdoctoral research fellow in the Faculty of Law at McGill University, Montréal. During the course of her doctoral research, she was a visiting scholar at Columbia Law School, a trainee in the Cabinet of Judge Christopher Vajda at the CJEU and worked on various projects alongside numerous international organisations including the Hague Institute for the Internationalisation of the Law. She is currently a member of the Academic Research Panel of Blackstone Chambers, London, and of the Abusive Lending Working Group of the Open Society Foundation’s Justice Initiative. Her research interests are in the areas of EU law, private international law and civil procedure; she has a particular interest in fundamental rights protections of vulnerable persons, transnational private and public regulation, and legal theory, areas in which she has published consistently. She has worked for a number of years in these fields as a researcher for a number of organisations, national governments and international institutions (including the European Commission), and has taught in Luxembourg, the Netherlands, Germany and the UK.
Back to the schedule
Mathias Niepert
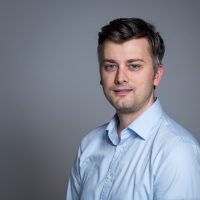
Date and Time:
- Tuesday, September 21, 2021, at 11:30 CEST
- To listen to this lecture, make sure you have registered for the Tuesday Session.
Title:
Fairness and Bias in Machine Learning
Abstract:
Machine learning is increasingly used for decision-making in several areas such as healthcare and banking. While this provides several opportunities it also poses problems with respect to amplifying or even introducing harmful biases. The lecture’s aim is to provide an overview of notions of bias and fairness and machine learning and of recent work on mitigating and understanding these biases.
Bio:
Mathias is Manager of the Machine Learning group and Chief Research Scientist for AI at NEC Laboratories Europe. After obtaining his PhD in computer science from Indiana University, he was a postdoctoral research associate at the Allen School of Computer Science, University of Washington and a member of the Data and Web Science Research Group at the University of Mannheim. Mathias’s research interests include representation learning for graph-structured data, geometric deep learning and probabilistic graphical models. His group’s methods are concerned with learning, inducing, and leveraging relational structure with applications in vision, natural language processing, and the (bio-)medical domain.
Back to the schedule
Katharina Kinder-Kurlanda
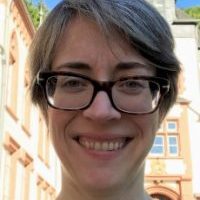
Date and Time:
- Wednesday, September 22, 2021, at 11:00 CEST
- To listen to this lecture, make sure you have registered for the Wednesday Session.
Title:
AI and Research Ethics – Theory & Practice
Abstract:
“Where, for example, anonymizing data, adopting pseudonyms, or granting or withholding consent makes no difference to outcomes for an individual, we had better be sure that the outcomes in question can be defended as morally and politically legitimate.”
(Barocas, Solon & Helen Nissenbaum: Big Data’s End Run around Anonymity and Consent” In: Book of Anonymity, edited by Anon Collective. Milky Way, Earth: punctum books (2021), p. 116-141.)
This mix between a lecture and a workshop addresses issues of ethical decision making in the individual NoBIAS research projects. Various ethical decision points arise in any research, particularly if social media data or other user-generated content are used. Research ethics issues in the use of social media data are closely related to various methodological and epistemological problems. Data-generating systems are, after all, designed and implemented to generate and hold very specific data that are not originally designed to be used as research data. By using internet platform data, we also become complicit in such platforms’ surveillance and in business practices that aim to generate value out of data. What is more, focusing on privacy no longer necessarily helps to address ethical concerns, or may even hide important issues.
Bio:
Katharina Kinder-Kurlanda is a Professor of Digital Culture at the Digital Age Research Center (D!ARC) at Klagenfurt University. She studied cultural anthropology, computer science and history in Tübingen and Frankfurt in Germany and received her Ph.D. from Lancaster University in the UK in 2009. Before coming to Klagenfurt, she was a team leader at the GESIS – Leibniz Institute for the Social Sciences. She works between disciplines, with the aim of building bridges, especially between the social sciences and computer science. Her research interests are new epistemologies for Big Data, algorithms in everyday life and work, data practices, data ethics, and social casual games.
Back to the schedule
Workshops*
- To sign up for a workshop, please click here.
*Workshops are for ESRs and Ph.D. Students from partner institutions only.
Back to the schedule
Organization and Contact
For questions and issues regarding the NoBias Summer School, please contact:
- Steffen Staab, University of Southampton & University of Stuttgart
- Sierra Kaiser, Interchange Forum for Reflecting on Intelligent Systems (SRF IRIS),
University of Stuttgart
Advisors
- Mayra Russo Botero, Leibniz University of Hannover
- Stephanie Law, University of Southampton
- Katharina Kinder-Kurlanda, University of Klagenfurt
- Eirini Ntoutsi, FU Berlin
A special thank you to the Interchange Forum for Reflecting on Intelligent Systems (SRF IRIS) for providing the online platform for the NoBias Summer School.